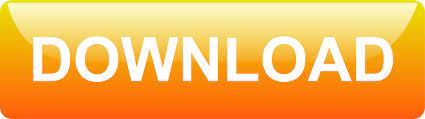
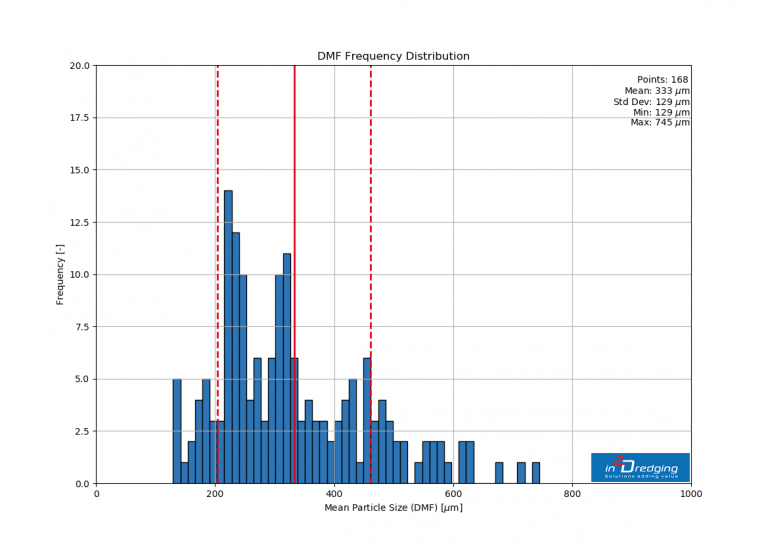
One solution here is to be much more stringent with “significance” levels, moving to P<0.001 or beyond, rather than P<0.05. w7 These false positive findings are the true products of data dredging, resulting from simply looking at too many possible associations. When a large number of associations can be looked at in a dataset where only a few real associations exist, a P value of 0.05 is compatible with the large majority of findings still being false positives. w6 The misinterpretation of a P<0.05 significance test as meaning that such findings will be spurious on only 1 in 20 occasions unfortunately continues. Data dredging is thought by some to be the major problem: epidemiologists have studies with a huge number of variables and can relate them to a large number of outcomes, with one in 20 of the associations examined being “statistically significant” and thus acceptable for publication in medical journals. It would seem wiser to attempt a better diagnosis of the problem before prescribing Le Fanu’s solution.
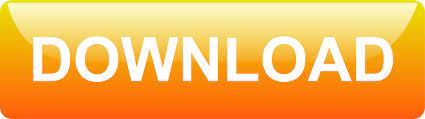